IoT Curator – April 2018
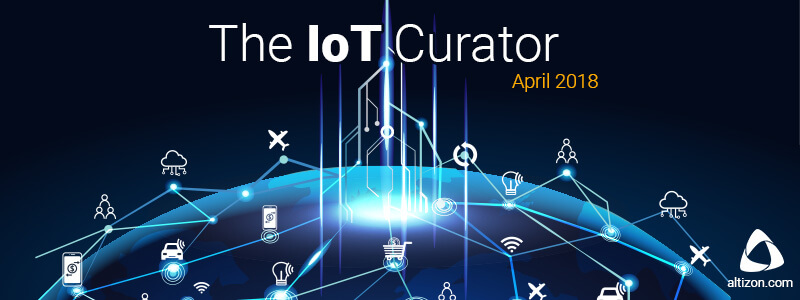
IoT Curator | Month of April buzzing with IIoT Trends – April 2018
Various events around the world kept Month of April buzzing with IIoT. We present you 3 links worth your time to know about whats happening in the world of Industrial Internet of things and events.
1. Hannover Messe 2018 – This is probably the biggest, largest, and longest IIoT or Industry40 event that takes place on the planet. I was talking to someone yesterday who just returned from HM2018 and showed me his mobile app tracking over 70 Kilometers walk in 7 days are the event. No kidding! It’s absolute extravaganza event when it comes to Industrial Automation and Technology. Here are some post-event glimpses, but this is a 12 mins summary video put together by Allen Behrens and Diago, its worth your time.
2. Frost & Sullivan’s take on major challenges for IIoT adoption in 2018 – F&S has come up with their observations on how the industry looks at IIoT solutions and what challenges currently prohibit the faster adoption. Original equipment manufacturers (OEMs), such as a pump, motor, and variable frequency drives (VFD) companies, want to leverage IIoT and bundle their products with IIoT products. F&S notes that these OEMs expects one-stop solution provider. However, there are not many such solution providers is one of the biggest challenges. Secondly, the overall cost of IIoT projects should be less than that of the assets to be monitored, then only it makes justifiable. Read the complete view here.
3. Transfer Learning – In standard supervised machine learning, we use sample or training data, i.e. a set of data points with known labels, and we build a model to learn the distinguishing properties that separate data points with different labels. This trained model can then be used to make label predictions for new data points. If we want to make predictions for another task (with different labels) in a different domain, we cannot use the model trained previously. We need to gather training data with the new task and train a separate model. Transfer learning provides a framework to leverage the already existing model (based on some training data) in a related domain. We can transfer the knowledge gained in the previous model to the new domain (and data). This article is worth your time as it gives a completely new perspective on ML.